Meet Jenia Jitsev at Max-Planck-Institute for Intelligent Systems
We are delighted to announce a talk by Jenia Jitsev, Jülich Supercomputing Centre and co-founder of Laion.
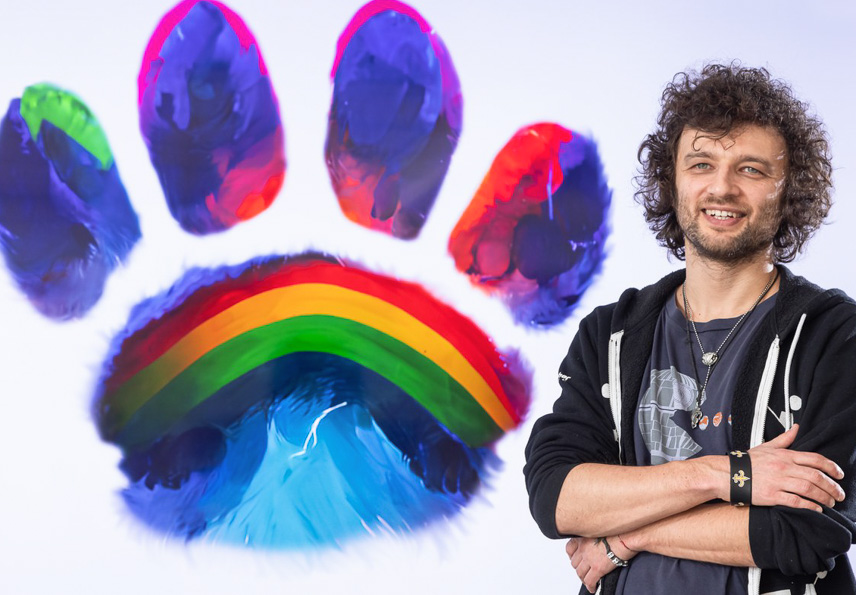
Details of the talk:
- Date: April 24, 2024
- Time: 11:00 a.m. - 12:00 p.m.
- Location: Ground-floor lecture hall - N0.0002, at Max Planck Institute for Intelligent Systems (Max-Planck-Ring 4, 72076 Tübingen)
Talk title: Open foundation models: reproducible science of transferable learning
Abstract: Recently, breakthroughs in strongly transferable learning was achieved by training models that use simple, generic losses and large amounts of generic, diverse web-scale data. Crucial for the progress was increasing pre-training scales, that is model, compute and dataset scales employed in the training. Derived scaling laws suggest that generalization and transferability improve when increasing scales hand in hand. Studying learning at such large scales is challenging, as it requires corresponding datasets at suffiently large scales to be available, sufficient compute resources to execute the training, while handling properly distributed training across thousands of compute nodes without suffering instabilities. We show how work done by LAION community made the whole pipeline for training strongly transferable multi-modal models of various kind (openCLIP, openFlamingo) - termed foundation models - fully open and reproducible. We show how important experiments necessary for studying such models, for instance those leading to scaling laws derivation, critically depend on open and reproducible nature of such pipelines. We conclude with outlook on studying next generation open multi-modal foundation models and datasets necessary for their creation.
Bio: Jenia Jitsev is computer scientist and neuroscientist, who is co-founder and scientific lead of LAION e.V, the German non-profit research organization committed to open science around large-scale foundation models (openCLIP, openFlamingo) and datasets (LAION-400M/5B, DataComp). He also leads Scalable Learning & Multi-Purpose AI (SLAMPAI) lab at Juelich Supercomputer Center of Helmholtz Association, Germany. His research happens in the overlap of machine learning and neuroscience, seeking to investigate learning as a generic process of incrementally building up a useful model of the surrounding world from available sensory observations and executed actions. He did his PhD with Frankfurt Institute for Advanced Studies (FIAS) on unsupervised learning in hierarchically organized recurrent networks of the visual cortex, and continued as postdoc with Max Planck Institute for Neurological Research in Cologne and Institute of Neuroscience and Medicine in Research Center Juelich, working on models of unsupervised and reinforcement learning in cortico-basal ganglia loops. In LAION and in his lab at Juelich Supercomputing Center, Dr. Jitsev current focus is on driving and democratizing research on scalable systems for generalist, transferable multi-modal learning, leading to foundation AI models capable of strong transfer with predictable behavior derived from corresponding scaling laws, and therefore easily adaptable to broad range of desired tasks and hardware resource settings. For his work, Dr. Jitsev received Best Paper Award at IJCNN 2012, Outstanding Paper Award at NeurIPS 2022 and Falling Walls Award for Scientific Breakthrough 2023.